
Information-theoretic analysis of deep identification systems
The vast majority of consumer goods are a target for counterfeiters. The advent of machine learning, including the deep neural networks, has encouraged the appearance of powerful simulation technologies capable to reproduce and generate complex images and textures. These tools are well known and available to the public. Therefore, it is important to develop technologies that allow to accurately and effectively detect counterfeits and verify the authenticity of products without any special equipment.
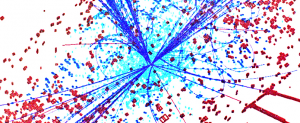
RODEM:Robust Deep Density Models
for High-Energy Physics and Solar Physics
RODEM is a SNSF/SINERGIA project fostering cooperation between high energy physicists, solar physicists and experts in machine learning in Switzerland to advance research methodologies in both fields.
A Sinergia research project funded by the Swiss National Science Foundation SNSF 2021-2024
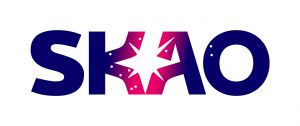
Astro Signals
Data & Science for the new generation of very larg radio-astronomy facilities.
An SNSF Sinergia project.
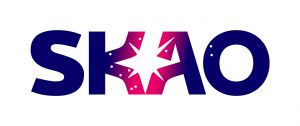
SERI
Link
Previous projects

Forensic Physical Object Identification
The main goal is to extend and further develop a new information-theoretic framework for physical object identification in the high-dimensional space of forensic features and apply it to practical systems. This goal will be achieved based on a novel interpretation of links between the encoding techniques, machine learning and forensic identification, which will be theoretically introduced and explored in this project.

Machine Learning based Analytics for Big Data in Astronomy
Astronomical observations produce a wealth of data in excess of several TB per day. Clearly, even a fraction of such data cannot be analyzed manually. In this project, we will investigate the use of big data analytics tools such as machine learning techniques applied to astronomical data. Specifically, we will consider observations of solar flares – magnetic eruptions that influence the whole solar system and cause space weather phenomena on Earth such as blackouts and problems in aircraft communication and GPS positioning.
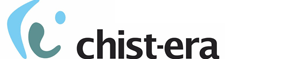
Identification for the Internet-of-Things
The IoT will contain a huge number of devices and objects that have very low or nonexistent processing and communication resources, coupled to a small number of high-power devices. The weakest devices, which are most ubiquitous, will not be able to authenticate themselves using cryptographic methods. Other important tasks in the IoT will be to verify if an object is authentic, or to identify an object. Our plan is to address these issues using Physical Unclonable Functions (PUFs). PUFs, and especially Quantum Readout PUFs, are ideally suited to the IoT setting because they allow for the authentication and identification of physical objects without requiring any crypto or storage of secret information. Furthermore, we foresee that back-end systems will not be able to provide security and privacy via cryptographic primitives due to the sheer number of IoT devices. Our plan is to address these problems using privacy-preserving database structures and algorithms with good scaling behaviour. Approximate Nearest Neighbour (ANN) search algorithms, which have remarkably good scaling behaviour, have recently become highly efficient, but do not yet have the right security properties and have not yet been applied to PUF data. Summarised in a nutshell, the project aims to improve the theory and practice of technologies such as PUFs and ANN search in the context of generic IoT authentication and identification scenarios.