- Physical object security
- Privacy Protection
- Image processing
- Compressive sensing
- Multimedia security
- Machine learning
- Computer vision
- Text detection
- Sparse Ternary Code
- Adversarial Machine Learning
Physical object security
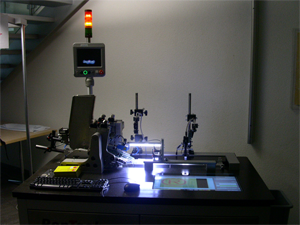
Physical object security
A video summary of technologies developed by the SIP group for physical object security
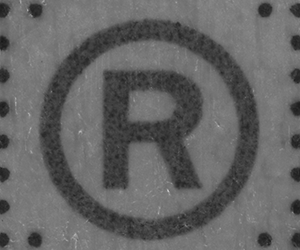
FAMOS
The first public optical PUF dataset for reproducible reserach
The forensic authentication microstructure optical set (FAMOS) is a dataset with 5000 unique microstructures from consumer packages for the development, testing and benchmarking of forensic identification and authentication technologies. All samples have been acquired 3 times with two different cameras giving 30.000 images in total.
@INPROCEEDINGS{Diephuis:2012:WIFS, author = {Sviatoslav Voloshynovskiy and Maurits Diephuis and Fokko Beekhof and Oleksiy Koval and Bruno Keel}, title = {Towards Reproducible results in authentication based on physical non-cloneable functions: The Forensic Authentication Microstructure Optical Set (FAMOS)}, year = {2012}, address = {Tenerife, Spain}, month = {December 2--5}, booktitle = {Proceedings of IEEE International Workshop on Information Forensics and Security} }
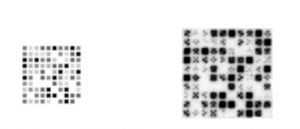
Security with printed bar codes
Link
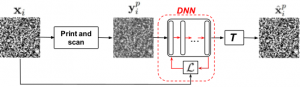
Clonability of anti-counterfeiting printable graphical codes: a machine learning approach
IEEE International Conference on Acoustics, Speech and Signal Processing (ICASSP) 2019
In recent years, printable graphical codes have attracted a lot of attention enabling a link between the physical and digital worlds, which is of great interest for the IoT and brand protection applications. The security of printable codes in terms of their reproducibility by unauthorized parties or clonability is largely unexplored. In this paper, we try to investigate the clonability of printable graphical codes from a machine learning perspective. The proposed framework is based on a simple system composed of fully connected neural network layers. The results obtained on real codes printed by several printers demonstrate a possibility to accurately estimate digital codes from their printed counterparts in certain cases. This provides a new insight on scenarios, where printable graphical codes can be accurately cloned
@inproceedings { Taran2019icassp, author = { Taran, Olga and Bonev, Slavi and Voloshynovskiy, Slava }, booktitle = { IEEE International Conference on Acoustics, Speech and Signal Processing (ICASSP) }, title = { Clonability of anti-counterfeiting printable graphical codes: a machine learning approach }, address = { Brighton, United Kingdom }, month = { May }, pages = { }, year = { 2019 } }

Banknote protection
PDFBibtex@inproceedings { Dewaele:2016:SPIE, author = { Thomas Dewaele, Maurits Diephuis, Taras Holotyak, Sviatoslav~Voloshynovskiy }, title = { Forensic authentication of banknotes on mobile phones }, booktitle = { Proceedings of SPIE Photonics West, Electronic Imaging, Media Forensics and Security V }, year = { 2016 }, month = { January, 14-18 }, address = { San Francisco, USA } }
SCA Privacy Protection Mechanism
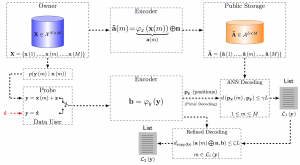
SCA Privacy-Preserving Identification: Fundamentals
Link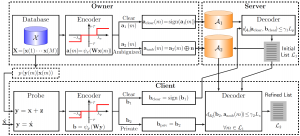
SCA Privacy-Preserving Outsourced Media Search
Link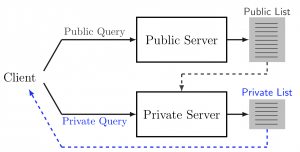
Privacy-Preserving Identification: Distributed Servers and Multiple Access Authorization
LinkSparse Ternary Code (STC)
Image processing
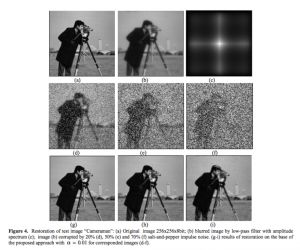
Image Restoration
Our research interests in inverse problems cover recovery from:
- highly distorted images that include blur and mixture noise originating from several distributions
- missed samples (non-uniform or sparse sampling) in both coordinate and Fourier domains
- quantized sparse sampling
- optimisation techniques suitable for high dimensional data machine-learning based methods
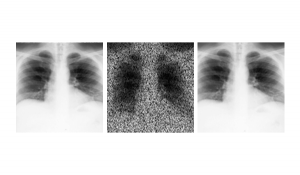
Image denoising
Gaussian noise
Misssed samples
Robust estimators
POCS
@article { Svolos:SP2005:IDEP, author = { Sviatoslav Voloshynovskiy and Oleksiy Koval and Thierry Pun }, title = { Image denoising based on the edge-process model }, journal = { Signal Processing }, year = { 2005 }, volume = { 85 }, pages = { 1950--1969 }, vgclass = { refpap }, vgproject = { watermarking }, number = { 10 }, month = { October } }
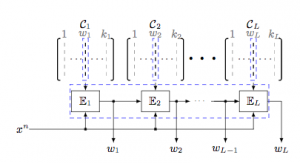
Face image compression
We study several schemes for multi-layer representation of images. The problem is first treated from an information-theoretic viewpoint where we analyze the behavior of different sources of information under a multi-layer data compression framework and compare it with a single-stage (shallow) structure. We then consider the image data as the source of information and link the proposed representation scheme to the problem of multi-layer dictionary learning for visual data. For the current work we focus on the problem of image compression for a special class of images where we report a considerable performance boost in terms of PSNR at high compression ratios in comparison with the JPEG2000 codec.
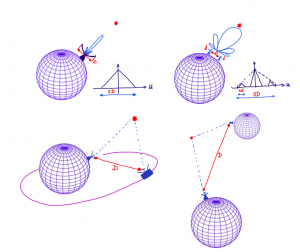
Sparse antenna arrays and radio interferometry imaging
The Swiss SKA day at EPFL
In this project, we intestigate the possibility of image recovery from sparsely sampled data in spatial frequency domain. We study different geomentry of antenna arrays coupled with the non-liner properties of reconstruction algorithms.
On-fly learning compressive sensing
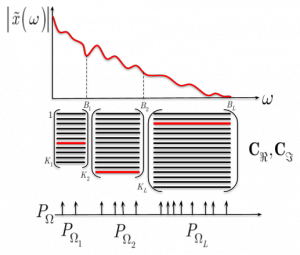
Injecting Image Priors into Learnable Compressive Subsampling
Many medical (computerized tomography, magnetic resonance imaging) and astronomy imaging problems (Square Kilometre Array), spectroscopy and Fourier optics attempt at reconstructing high quality images in the pixel domain from a limited number of samples in the frequency domain. In this paper, we extend the problem formulation of learnable compressive subsampling that focuses on the learning of the best sampling operator in the Fourier domain adapted to spectral properties of training set of images. We formulate the problem as a reconstruction from a finite number of sparse samples with a prior learned from the external dataset or learned on-fly for the image to be reconstructed. The proposed methods are tested on diverse datasets covering facial images, medical and multi-band astronomical applications using the mean square error and SSIM as a perceptual measure of reconstruction. The obtained results demonstrate some interesting properties of proposed methods that might be of interest for future research and extensions.
@inproceedings { Taran2018eusipco, author = { Ferrari, Martino and Taran, Olga and Holotyak, Taras and Egiazarian, Karen and Voloshynovskiy, Slava }, booktitle = { 26th European Signal Processing Conference (EUSIPCO) }, title = { Injecting Image Priors into Learnable Compressive Subsampling }, address = { Rome, Italy }, pages = { }, month = { September }, year = { 2018 } }
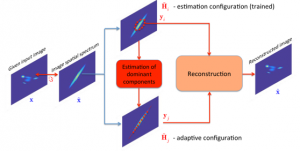
Robust image restoration matched with Adaptive Aperture Formation in radar imaging systems with sparse antenna arrays
The paper presents a complex approach to radar imaging system development. The approach consists of two main stages. The first stage is adaptive aperture formation and the second one is robust adaptive image restoration. The use of adaptive aperture formation strategy makes possible to estimate the principle image components in spatial frequency domain and increase the reliability of received data. The robust adaptive image restoration allows to compensate the blurring effect of sparse aperture in the presence of mixed noise (i.e. Gaussian and impulse). The efficiency of the proposed approach is investigated on numerous test examples.
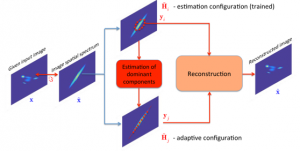
Adaptive aperture formation matched with radiometry image spatial spectrum
The new approach to the radiometry image formation based on the matching of image characteristics with aperture synthesis is proposed. Quatitive analyze and comparison with conventional methods of radiometry imaging are performed.
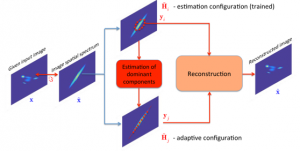
SKA machine learning perspectves for imaging, processing and analysis
with contribution of:
D. Kostadinov, S. Ferdowsi, M. Diephuis, O. Taran and T. Holotyak
Multimedia security
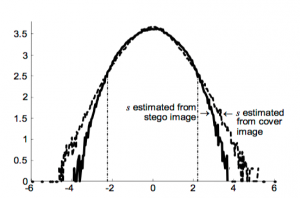
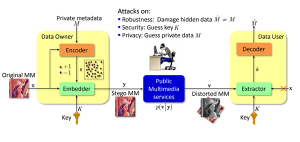
Robust watermarking for copyright protection
Link
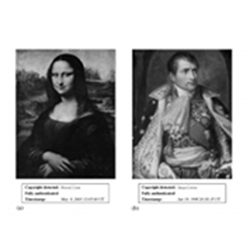
Content authentication and tamper proofing based data hiding
PDF
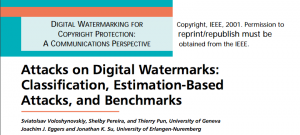
Watermarking attacks and benchmarking
PDF
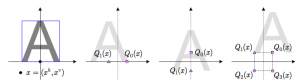
Text watermarking
PDFBibtex@inproceedings { Villan:SPIE2007:RH, author = { Renato Vill{\'a}n and Sviatoslav Voloshynovskiy and Oleksiy Koval and Fr\'{e}d\'{e}ric Deguillaume and Thierry Pun }, title = { Tamper-proofing of Electronic and Printed Text Documents via Robust Hashing and Data-Hiding }, booktitle = { Proceedings of {SPIE-IS{\&}T} Electronic Imaging 2007, Security, Steganography, and Watermarking of Multimedia Contents IX }, year = { 2007 }, vgclass = { refpap }, vgproject = { watermarking }, address = { San Jose, USA }, month = { 28 Jan. -- 1 Feb. }, abstract = { In this paper, we deal with the problem of authentication and tamper-proofing of text documents that can be distributed in electronic or printed forms. We advocate the combination of robust text hashing and text data-hiding technologies as an efficient solution to this problem. First, we consider the problem of text data-hiding in the scope of the Gel'fand-Pinsker data-hiding framework. For illustration, two modern text data-hiding methods, namely color index modulation (CIM) and location index modulation (LIM), are explained. Second, we study two approaches to robust text hashing that are well suited for the considered problem. In particular, both approaches are compatible with CIM and LIM. The first approach makes use of optical character recognition (OCR) and a classical cryptographic message authentication code (MAC). The second approach is new and can be used in some scenarios where OCR does not produce consistent results. The experimental work compares both approaches and shows their robustness against typical intentional/unintentional document distortions including electronic format conversion, printing, scanning, photocopying, and faxing. } }
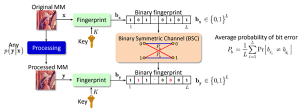
Content fingerprinting
Link
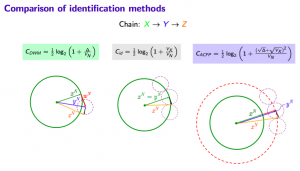
Active Content Fingerprinting
Link
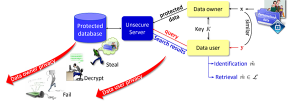
Privacy preserving search and authentication
Link
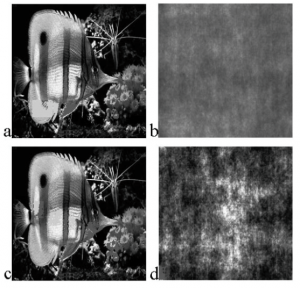
Visual scrambling robust to distortions
PDFLinkBibtex@article { , grytskiv:1998:facta title = { Cryptography and steganography of video information in modern communications }, author = { Z. Grytskiv and Sviatoslav Voloshynovskiy and Yuriy Rytsar }, journal = { Facta Universitatis }, year = { 1998 }, volume = { 11 }, number = { 1 }, pages = { 115--125 }, vgclass = { refpap }, vgproject = { watermarking } }
Machine learning
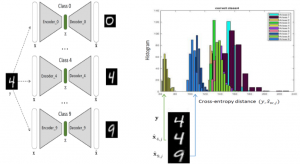
Classification by Re-generation: Towards Classification Based on Variational Inference
As Deep Neural Networks (DNNs) are considered the state-of-the-art in many classification tasks, the question of their semantic generalizations has been raised. To address semantic interpretability of learned features, we introduce a novel idea of classification by re-generation based on variational autoencoder (VAE) in which a separate encoder-decoder pair of VAE is trained for each class. Moreover, the proposed architecture overcomes the scalability issue in current DNN networks as there is no need to re-train the whole network with the addition of new classes and it can be done for each class separately. We also introduce a criterion based on KullbackLeibler divergence to reject doubtful examples. This rejection criterion should improve the trust in the obtained results and can be further exploited to reject adversarial examples.
@inproceedings { Rezaeifar2018eusipco, author = { Shideh Rezaeifar and Olga Taran and Slava Voloshynovskiy }, booktitle = { 26th European Signal Processing Conference (EUSIPCO) }, title = { Classification by Re-generation: Towards Classification Based on Variational Inference }, address = { Rome, Italy }, pages = { }, month = { September }, year = { 2018 } }
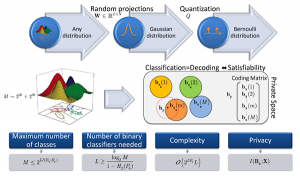
Multiclass classification
Description
@article { Voloshynovskiy:2011:theoretic, title = { Theoretic Multiclass Classification Based on Binary Classifiers }, author = { Sviatoslav Voloshynovskiy and Oleksiy Koval and Fokko Beekhof and Taras Holotyak }, journal = { Signal Processing Systems }, year = { 2011 }, volume = { 65 }, pages = { 413-430 }, note = { (accepted) } }
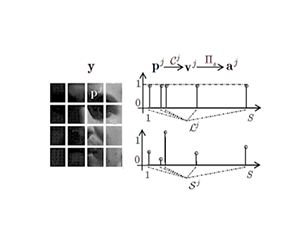
Adversarial Machine Learning
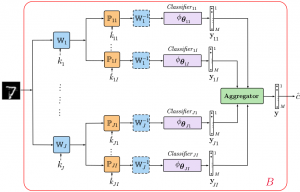
Defending against adversarial attacks by randomized diversification
The IEEE Conference on Computer Vision and Pattern Recognition (CVPR) 2019
The vulnerability of machine learning systems to adversarial attacks questions their usage in many applications. In this paper, we propose a randomized diversification as a defense strategy. We introduce a multi-channel architecture in a gray-box scenario, which assumes that the architecture of the classifier and the training data set are known to the attacker. The attacker does not only have access to a secret key and to the internal states of the system at the test time. The defender processes an input in multiple channels. Each channel introduces its own randomization in a special transform domain based on a secret key shared between the training and testing stages. Such a transform based randomization with a shared key preserves the gradients in key-defined sub-spaces for the defender but it prevents gradient back propagation and the creation of various bypass systems for the attacker. An additional benefit of multi-channel randomization is the aggregation that fuses soft-outputs from all channels, thus increasing the reliability of the final score. The sharing of a secret key creates an information advantage to the defender. Experimental evaluation demonstrates an increased robustness of the proposed method to a number of known state-of-the-art attacks.
@inproceedings { Taran2019cvpr, author = { Taran, Olga and Rezaeifar, Shideh and Holotyak, Taras and Voloshynovskiy, Slava }, booktitle = { The IEEE Conference on Computer Vision and Pattern Recognition (CVPR) }, title = { Defending against adversarial attacks by randomized diversification }, address = { Long Beach, USA }, month = { June }, pages = { }, year = { 2019 } }
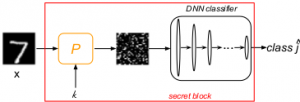
Bridging machine learning and cryptography in defence against adversarial attacks
Workshop on Objectionable Content and Misinformation (WOCM), ECCV 2018
Most of the deep learning architectures are vulnerable to so called adversarial examples. This questions the security of deep neural networks (DNN) for many security- and trust-sensitive domains. The majority of the proposed existing adversarial attacks are based on the differentiability of the DNN cost function. Defence strategies are mostly based on machine learning and signal processing principles that either try to detect-reject or filter out the adversarial perturbations and completely neglect the classical cryptographic component in the defence. In this work, we propose a new defence mechanism based on the second Kerckhoffs’s cryptographic principle which states that the defence and classification algorithm are supposed to be known, but not the key. To be compliant with the assumption that the attacker does not have access to the secret key, we will primarily focus on a gray-box scenario and do not address a white-box one.
@inproceedings { Taran2018WOCM, author = { Olga Taran and Shideh Rezaeifar and Slava Voloshynovskiy }, booktitle = { Workshop on Objectionable Content and Misinformation (WOCM), ECCV2018 }, title = { Bridging machine learning and cryptography in defence against adversarial attacks }, address = { Munich, Germany }, pages = { }, month = { September }, year = { 2018 } }
Computer vision
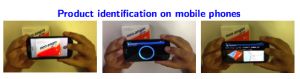
Object recognition on mobile phones
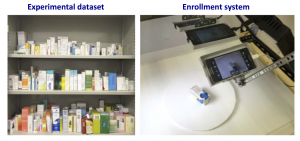
Database of real objects acquired by mobile phones
Ongoing project
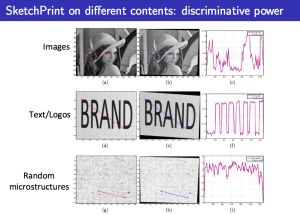
Feature extraction for computer vision
Link
Real time multi-language text detection
Text detection, projective transforms, real time, mobile app
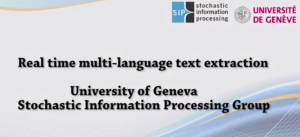
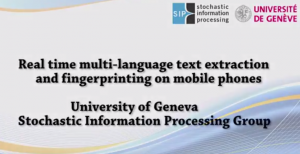
Text extraction and fingerprinting on phone
Demo on:
- pharmaceutical packages;
- licence plates;
- invoices and bills