Publications
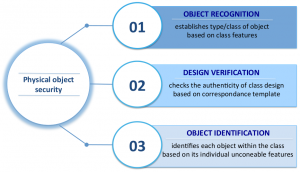
Digital Security for Physical World
Innovative solutions for physical object protection developed by the SIP group.
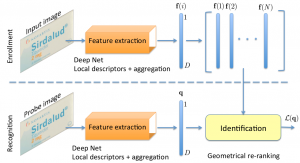
PharmaPack: mobile fine-grained recognition of pharma packages
We consider the problem of fine-grained physical object recognition and introduce a dataset PharmaPack containing 1000 unique pharma packages enrolled in a controlled environment using consumer mobile phones as well as several recognition sets representing various scenarios. For performance evaluation, we extract two types of recently proposed local feature descriptors and aggregate them using popular tools. All enrolled raw and pre-processed images, extracted and aggregated descriptors are made public to promote reproducible research. To evaluate the baseline performance, we compare the methods based on aggregation of local descriptors with methods based on geometrical matching.
@inproceedings { Taran:2017:EUSIPCO, author = { Olga Taran and Shideh Rezaeifar and Oscar Dabrowski and Jonathan Schlechten and Taras Holotyak and Slava Voloshynovskiy }, title = { PharmaPack: mobile fine-grained recognition of pharma packages }, booktitle = { European Signal Processing Conference (EUSIPCO) }, month = { 28 August - 2 September }, year = { 2017 }, address = { Kos, Grece } }
Fine-grained identification of pharmaceutical products based on local descriptors
In this work, the problem of fine-grained identification of pharmaceutical products is addressed. A large database of images of pharma packages acquired by modern mobile phones was created. The contribution of this thesis encompasses the development of 2 different approaches based on local descriptors and the investigation of the identification performance of these methods. Moreover, our contribution entails the setup and creation of a new database of images suitable for testing fine-grained recognition algorithms, which we hope can benefit to the computer vision community.
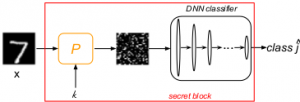
Bridging machine learning and cryptography in defence against adversarial attacks
Most of the deep learning architectures are vulnerable to so called adversarial examples. This questions the security of deep neural networks (DNN) for many security- and trust-sensitive domains. The majority of the proposed existing adversarial attacks are based on the differentiability of the DNN cost function. Defence strategies are mostly based on machine learning and signal processing principles that either try to detect-reject or filter out the adversarial perturbations and completely neglect the classical cryptographic component in the defence. In this work, we propose a new defence mechanism based on the second Kerckhoffs’s cryptographic principle which states that the defence and classification algorithm are supposed to be known, but not the key. To be compliant with the assumption that the attacker does not have access to the secret key, we will primarily focus on a gray-box scenario and do not address a white-box one.
@inproceedings { Taran2018WOCM, author = { Olga Taran and Shideh Rezaeifar and Slava Voloshynovskiy }, booktitle = { Workshop on Objectionable Content and Misinformation (WOCM), ECCV2018 }, title = { Bridging machine learning and cryptography in defence against adversarial attacks }, address = { Munich, Germany }, pages = { }, month = { September }, year = { 2018 } }
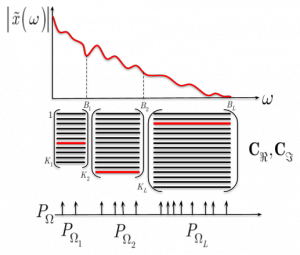
Injecting Image Priors into Learnable Compressive Subsampling
Many medical (computerized tomography, magnetic resonance imaging) and astronomy imaging problems (Square Kilometre Array), spectroscopy and Fourier optics attempt at reconstructing high quality images in the pixel domain from a limited number of samples in the frequency domain. In this paper, we extend the problem formulation of learnable compressive subsampling that focuses on the learning of the best sampling operator in the Fourier domain adapted to spectral properties of training set of images. We formulate the problem as a reconstruction from a finite number of sparse samples with a prior learned from the external dataset or learned on-fly for the image to be reconstructed. The proposed methods are tested on diverse datasets covering facial images, medical and multi-band astronomical applications using the mean square error and SSIM as a perceptual measure of reconstruction. The obtained results demonstrate some interesting properties of proposed methods that might be of interest for future research and extensions.
@inproceedings { Taran2018eusipco, author = { Ferrari, Martino and Taran, Olga and Holotyak, Taras and Egiazarian, Karen and Voloshynovskiy, Slava }, booktitle = { 26th European Signal Processing Conference (EUSIPCO) }, title = { Injecting Image Priors into Learnable Compressive Subsampling }, address = { Rome, Italy }, pages = { }, month = { September }, year = { 2018 } }
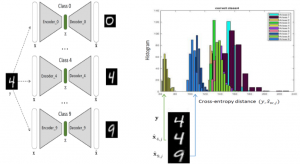
Classification by Re-generation: Towards Classification Based on Variational Inference
As Deep Neural Networks (DNNs) are considered the state-of-the-art in many classification tasks, the question of their semantic generalizations has been raised. To address semantic interpretability of learned features, we introduce a novel idea of classification by re-generation based on variational autoencoder (VAE) in which a separate encoder-decoder pair of VAE is trained for each class. Moreover, the proposed architecture overcomes the scalability issue in current DNN networks as there is no need to re-train the whole network with the addition of new classes and it can be done for each class separately. We also introduce a criterion based on KullbackLeibler divergence to reject doubtful examples. This rejection criterion should improve the trust in the obtained results and can be further exploited to reject adversarial examples.
@inproceedings { Rezaeifar2018eusipco, author = { Shideh Rezaeifar and Olga Taran and Slava Voloshynovskiy }, booktitle = { 26th European Signal Processing Conference (EUSIPCO) }, title = { Classification by Re-generation: Towards Classification Based on Variational Inference }, address = { Rome, Italy }, pages = { }, month = { September }, year = { 2018 } }